Design of Experiments (DoE) is a set of statistical and engineering methods that enables the planning, execution, and analysis of experiments in a way that maximizes the amount of knowledge gained while minimizing testing resources.
With DoE, engineers can thoroughly investigate the effects of various variables on process outcomes and identify key factors that influence system performance. The historical foundation of this method dates back to the work of Ronald Fisher β a pioneer in statistics β who in the early 20th century introduced a systematic approach to experimentation.
Since then, DoE has evolved by incorporating new techniques and tools such as Taguchi methods and full factorial designs, making it an invaluable resource for optimizing manufacturing processes and conducting research and development.
Fundamental Principles of Design of Experiments
At the core of DoE is the selection of relevant variables (factors) and defining their levels β typically two levels are used: high (+1) and low (β1). This approach allows the creation of full factorial designs, in which all possible combinations of factor settings are tested.
This enables engineers not only to assess the impact of individual variables but also to identify interactions between them. Unlike traditional methods that test one variable at a time (known as OFAT β One Factor At a Time), DoE makes it possible to detect synergistic or antagonistic effects among variables.
Single-factor methods often fail to reveal complex dependencies and can produce misleading results by ignoring interactions, which makes DoE significantly more efficient and reliable.
Methods and Techniques Used in Design of Experiments
One of the key tools in DoE is the use of orthogonal arrays. These are designed to ensure a balanced data set β each level of each variable appears an equal number of times β which enables the independent evaluation of each factor’s impact.
These techniques minimize aliasing (confounding), which occurs when the effects of two variables overlap, making it difficult to distinguish their individual impacts.
Full factorial experiments involve testing all combinations of factor levels. This is feasible with a small number of variables, but the number of required runs increases exponentially as more factors are added. For this reason, engineers often turn to alternative approaches, such as Taguchi methods, which reduce the number of tests while preserving valuable information about the system.
Another essential technique in DoE is variable coding β assigning values of β1 and +1 for low and high levels respectively. This produces a symmetric design that simplifies statistical analysis and interpretation of results.
Interaction analysis is also crucial. Properly designed experiments allow engineers to determine whether variables act independently or jointly affect outcomes. For instance, in optimizing a production process, factors like time, temperature, or pressure may have effects on their own, but may also interact in non-linear ways, impacting overall efficiency. Avoiding aliasing is therefore key to drawing accurate conclusions.
Practical Applications of DoE
DoE is widely used across various engineering sectors. In the automotive industry, it allows for the optimization of manufacturing processes, resulting in improved product quality and cost reduction.

For example, when optimizing parameters in plastic injection molding, engineers can investigate the impact of variables such as:
-
injection speed,
-
injection pressure,
-
mold temperature,
-
holding time.
DoE enables a reduction in the number of required tests (e.g., down to 12 runs in a full factorial experiment for selected variables). It also allows engineers to identify key factors, analyze interactions between them, and develop predictive models.
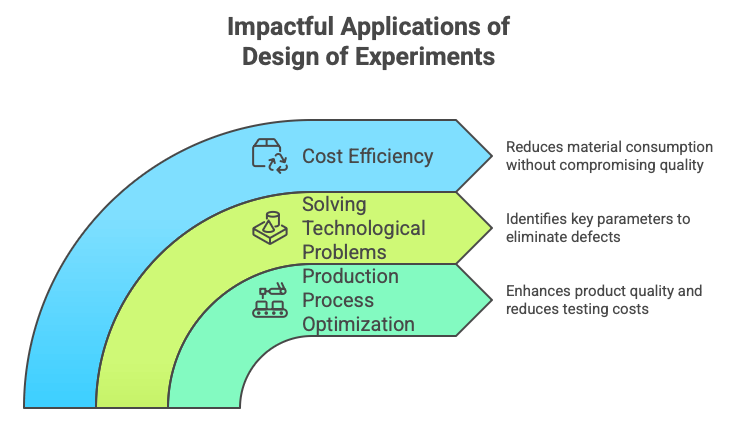
This makes it possible to determine the optimal process settings and even predict results for untested parameter combinations.
Another example is the use of DoE to eliminate undesirable visual effects in decorative components. In a project involving the production of vehicle cabin panels, engineers observed a βorange peelβ surface defect. Using DoE, they identified several process parameters β such as carrier temperature, press temperature, pressure force, and lamination time β that potentially contributed to the defect.
By testing each parameter at two levels in a full factorial experiment, they were able to pinpoint the exact combination that significantly reduced the visual defect. Statistical analysis confirmed that the optimal settings eliminated the issue entirely. This illustrates how DoE can quickly optimize processes, even with limited resources.
Case Study: Adhesive Consumption Optimization
A third case involved optimizing an adhesive application process, with the primary goal of reducing adhesive use without compromising bond quality. Using the DoE methodology, engineers analyzed the effects of five key parameters:
-
application speed,
-
application pressure,
-
temperature,
-
application time,
-
mixing intensity.
Each was tested at two levels. A full factorial design helped determine the most efficient parameter settings, which led to a 35% reduction in adhesive usage while maintaining comparable peel strength β critical for durability and product quality. These results translated into significant material cost savings.
Benefits of Implementing Design of Experiments
The main advantages of using DoE are efficiency and resource savings. The method provides a comprehensive understanding of a process using a minimal number of tests. This enables the creation of accurate predictive models that can forecast system behavior under various conditions without testing every possible combination.
By optimizing production processes through DoE, organizations can implement technological improvements faster, reduce costs, and gain competitive advantages.
Additionally, DoE helps engineers better understand complex systems and processes, which is crucial in the automotive sector where even small improvements can lead to significant cost savings and quality enhancements.
Engineers using DoE gain a powerful tool for systematically analyzing how process parameters affect results β leading to informed, data-driven decisions.
Summary and Conclusions
Design of Experiments is much more than a testing method β itβs a mindset that fosters deep understanding of complex systems. DoE enables engineers to identify key variables, analyze their interactions, and develop predictive models that support process optimization.
The implementation of DoE brings tangible benefits: cost savings, improved research efficiency, and faster adoption of technological innovations.
For companies in the automotive and other manufacturing industries, DoE can be a true competitive edge. Rather than relying on trial-and-error methods, engineers can systematically study the combined effects of multiple variables β resulting in more precise and impactful design decisions.
Author: RafaΕ Sobczak