Artificial intelligence (AI) plays an increasingly important role in quality management and quality engineering, with forecasts indicating it will revolutionize most quality-related processes in the coming years. There’s already a noticeable difference between companies quickly implementing AI and those still relying on traditional methods.
For quality engineers, AI is becoming a tool that enables easier data processing and analysis, contributing to shorter execution times for routine, repetitive tasks and supporting better decision-making.
Key Findings
- Artificial Intelligence is transforming quality management: 70-75% of quality control processes in the automotive industry are expected to be automated by AI soon, leading to faster defect detection and reduced complaint rates.
- Cost reduction: Some corporations are starting to report up to 45% reduction in quality control costs and return on investment often exceeding 35% in the first year through the use of intelligent data analysis tools.
- Time reduction: Algorithms allow for a 60-70% reduction in analysis and reporting time, enabling engineers to focus on projects that increase competitiveness.
- Multidimensional transformation: AI effectively supports root cause analysis, automates documentation assessment, and enables intelligent customer communication, although its implementation requires building appropriate competencies and acceptance among employees to fully utilize the synergy between algorithms and human experience.
Brief History and Evolution of AI in Quality
The first AI concepts date back to the 1950s when Alan Turing asked if machines could think. The term “artificial intelligence” was introduced in 1956, and subsequent decades brought expert system development. From the 1980s, these solutions were increasingly combined with quality engineering, enabling faster data analysis and decision support in control processes.
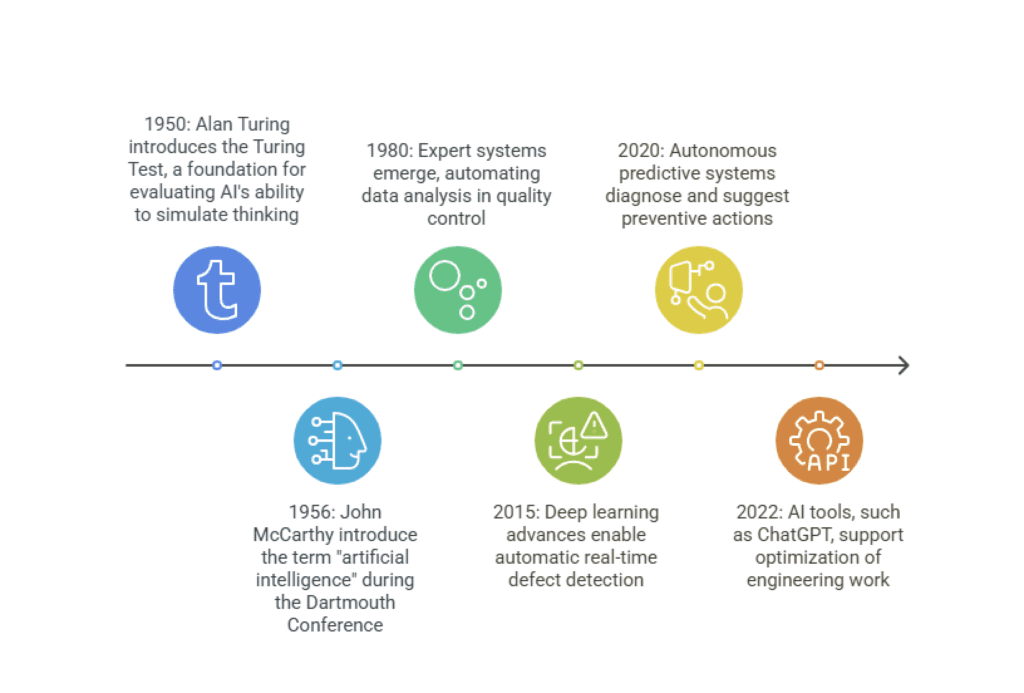
Recent years marked a breakthrough with AI tools becoming available to a wide range of users. Currently, solutions supporting daily engineering duties dominate, such as report automation, root cause analysis support, and rapid customer documentation processing.
Daily Applications in Quality Department
While media often highlights “spectacular” implementations, the transformation also happens at the quality engineer’s daily practice level. Analyses show that routine tasks like report preparation and customer requirements review consume significant work time (up to 60-70%). Basic AI tool implementation allows:
- reducing analysis time,
- automating of repetitive reports,
- speeding up production data deviation identification,
- improving business contact efficiency.
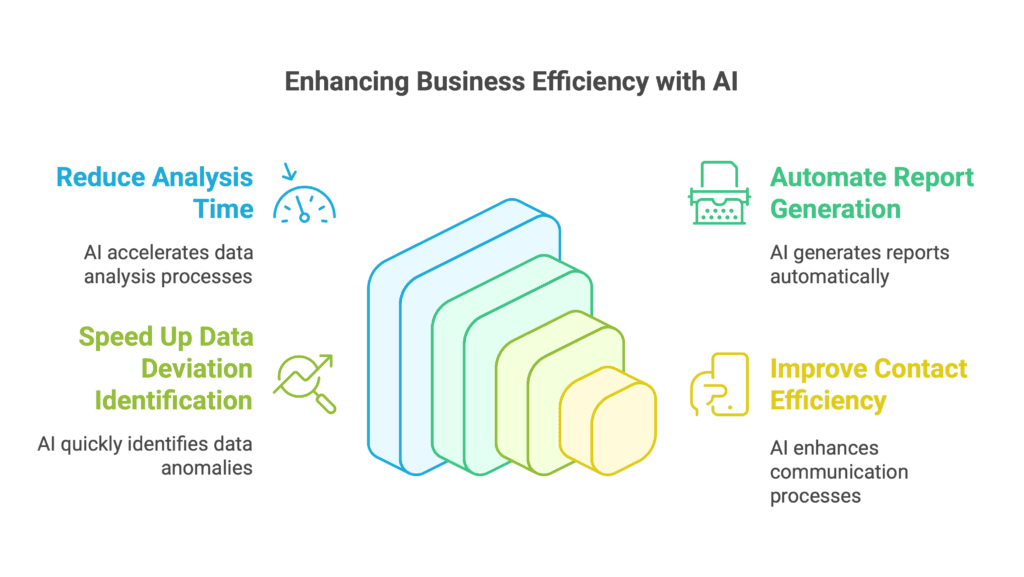
This enables quality engineers to focus on strategic tasks like planning new testing methods or implementing quality improvement projects.
Case Study 1: Customer Requirements Analysis Automation
Challenge
Growing documentation volume and increasing customer requirements cause engineers to lose time on manual data analysis. Additionally, specification and report checking increases the risk of missing important information, potentially leading to delays and affecting deadlines and costs.
Solution
Implementing AI tools processing PDF or text documents, classifying requirements by topic, competency, and risk. Algorithms prepare summaries and indicate key areas, allowing engineers to quickly identify priorities and share findings with the team. Tools like NotebookLM, Claude AI, or ChatGPT support these processes.
Results
- 60% analysis time reduction through automatic categorization.
- Reduced documentation review error risk.
- Focus on strategic tasks, improving response and customer satisfaction.
Case Study 2: Improving 8D Root Cause Analysis Effectiveness and Efficiency
Challenge
8D analysis requires cooperation between experts from different departments, making meeting organization and data collection difficult. Limited resources and lack of time for critical hypothesis analysis often delay reaching conclusions.
Solution
AI automates data analysis, generates assumption verification questions, and suggests solutions based on previous failures. This helps even less experienced team members quickly understand the problem and evaluate possible actions.
Results
- Reduced meeting numbers through better AI-prepared materials.
- Faster root cause identification through automatic data analysis.
- Team competency improvement through AI recommendations access.
Case Study 3: Communication in International Environment Using NVC
Challenge
Language and cultural differences hinder cooperation between quality engineers and foreign clients and suppliers, leading to communication misunderstandings, particularly in email correspondence.
Solution
Implementing NVC (Nonviolent Communication) models in AI language models enables quick email message formatting, resulting in much more effective communication.
Results
- Reduced correspondence misinterpretation.
- Better cooperation through more effective communication.
- Higher customer satisfaction through transparency and empathy in contacts.
Implementation Lessons
Companies successful in implementing AI in quality follow several key principles:
- Focus on specific challenge: Choose area with highest improvement potential (e.g., ISO or IATF requirements, complaint reduction, 8D analysis).
- Act in stages: Pilot in one department, expand solution to other areas only after achieving measurable results.
- Ensure data security: Besides industry standards (e.g., IATF 16949), remember proper encryption and data management, especially cloud-stored data.
- Develop team competencies: When implementing AI, ensure quality engineers have access to data analysis and basic programming training.
- Share successes: Communicate AI effects and benefits across company to gain support from various departments and management.
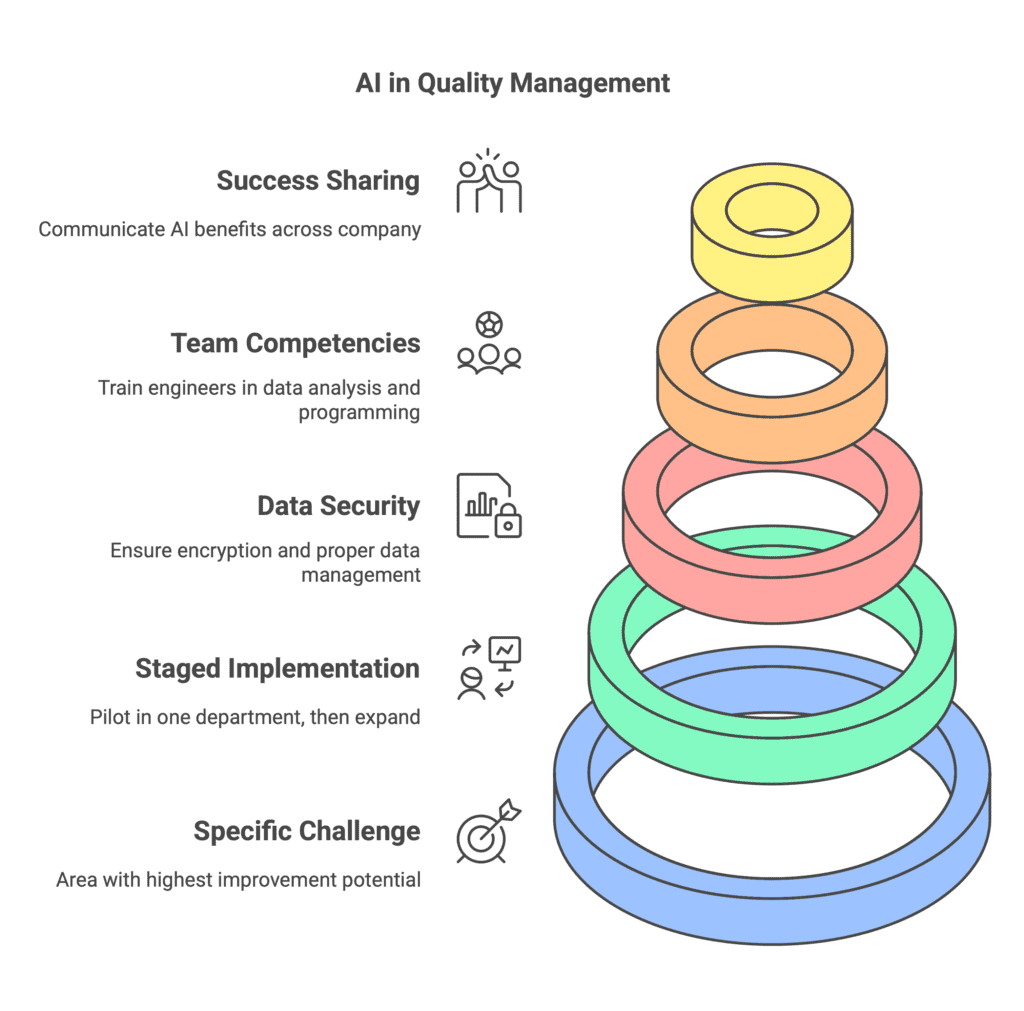
Challenges Related to AI Implementation
Although artificial intelligence brings numerous benefits, implementing it in quality management also comes with challenges that should be considered to ensure an effective and well-thought-out transformation process:
- Implementation Costs. The implementation of advanced AI systems requires significant financial investment, both for purchasing the technology and integrating it with existing processes. For many companies, especially small and medium-sized enterprises, this can be a barrier to entry.
- Lack of Skills. AI implementation often requires retraining current employees or hiring specialists in data analysis and programming. The shortage of such skills in the job market can delay the implementation process.
- Resistance to Change. Employees accustomed to traditional working methods may resist new technologies. Therefore, proper change management and team education are crucial to build trust in AI.
- Algorithm Dependency. Over-reliance on AI systems carries the risk of losing control over processes. It’s important that humans continue to play a key role in decision-making and system supervision, especially in situations requiring intuition or experience.
Taking these challenges into account allows for better preparation for implementations and minimizes the risk of failure while maximizing the benefits of using artificial intelligence in quality management.
Future and Key Trends
By 2030, AI will become a key quality tool, especially in highly automated sectors like automotive. Algorithms will independently analyze huge datasets and predict failures, significantly improving preventive action effectiveness.
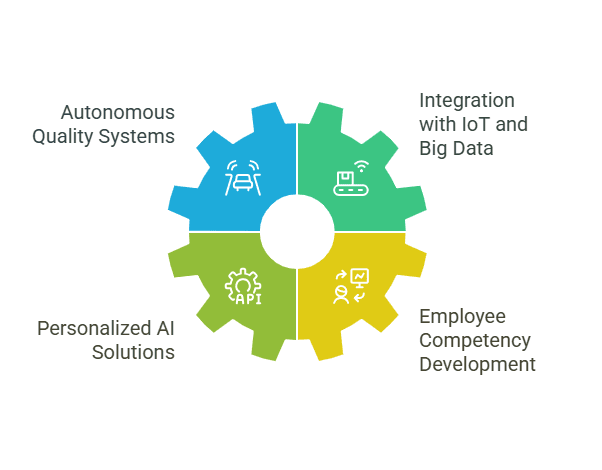
Autonomous Quality Management Systems
- AI will detect deviations in real-time and propose corrective actions.
- Quality engineer will transition to advisory role, verifying algorithm recommendations.
Integration with Other Technologies
- IoT and Big Data connection will enable better supply chain coordination.
- Comprehensive platforms will automate data analysis and improve manufacturing process.
Personalized AI Solutions
- Companies of various sizes will gain tailored systems with lower cost and high efficiency.
- Multifunctional AI assistants will replace traditional applications, consolidating analysis and decision support.
Employee Competency Development
- Training combining quality knowledge and advanced data analysis grows in importance.
- Employee role will focus on strategic information use, ensuring competitive advantage.
Summary: Future Belongs to People, Not Machines
Although artificial intelligence changes daily task execution, quality engineer still directs changes, sets priorities, and ensures business goal alignment. Key to effective AI in Quality Management use is skillfully combining algorithms with human knowledge and experience. This enables teams to respond faster to challenges, implement innovations, and spend more time on activities truly increasing company competitiveness.
Success Key Lies in Adaptation
Advantage doesn’t always come from advanced infrastructure or larger capital, but from quick change response ability. Start with one optimization area and conduct several-week pilot. If results satisfy, gradually extend AI to other processes, scaling success across organization.
Cooperation and Complementary Competencies
AI frees team from most tedious tasks like reporting or large dataset analysis, while strengthening its potential. However, AI won’t replace human creativity and strategic thinking ability. This lets engineers focus on improvements, innovations, and building better contractor relationships.
Human Always at Center
Remember that quality expert – with experience, empathy, and skills – remains key element in process development and strategy shaping. This approach ensures technology supports people, not vice versa, building continuous improvement culture.
References:
Roland Berger, “Automotive Outlook 2040”, 2024
Qualityze, “AI in Quality: Revolutionizing the QMS Industry,” , 2024.
Datategy, “How AI Transforms Quality Control in Modern Manufacturing?,” 2024.
Quality Magazine, “Case Studies of AI for Superhuman Quality Control in Electronics,”, 2025.
ICert Global, “The Impact of Artificial Intelligence on Quality Management Systems,” , 2024.